Understanding AI Agents: A Comprehensive Guide to the Future of AI Implementation
Table of Contents
- What is an AI Agent and Why Does it Matter?
- The Capabilities and Value Logic of Agents
- A Classification System for AI Agents Based on Implementation
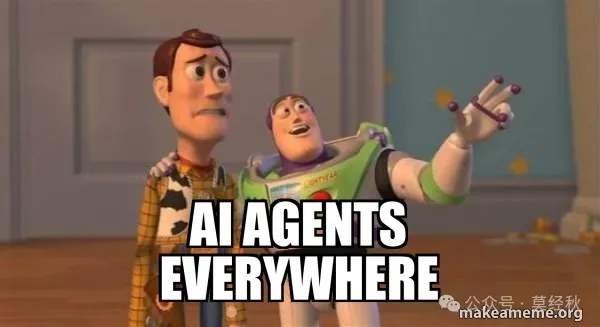
The ubiquitous nature of AI Agents in modern applications
1. What is an AI Agent and Why Does it Matter?
Due to extensive science fiction influence, people's imagination of AI often leaps directly to seamless integration into human work and life. Some even envision AI physically entering the real world, ready to either liberate or subjugate humanity. However, there remains a significant gap between research papers, code, data, and real-world applications.
At its core, AI is fundamentally a computational model - more broadly speaking, AI is a complex function formula y=f(x). If this formula exists in isolation, it's like an uncalled function method, serving no practical purpose. An Agent is the instantiation of these model methods, the practical implementation of models in application environments.
Historical Context
Long before ChatGPT sparked an industry revolution, agents were already widely used in various computational and simulation fields. For example:
- NPCs in games are agents, automatically selecting preset dialogues based on player behavior
- Simulated vehicles in road design are agents, running simple automation scripts
- Aircraft in flight simulators are agents, carrying flight dynamics models that sense the environment and output motion states
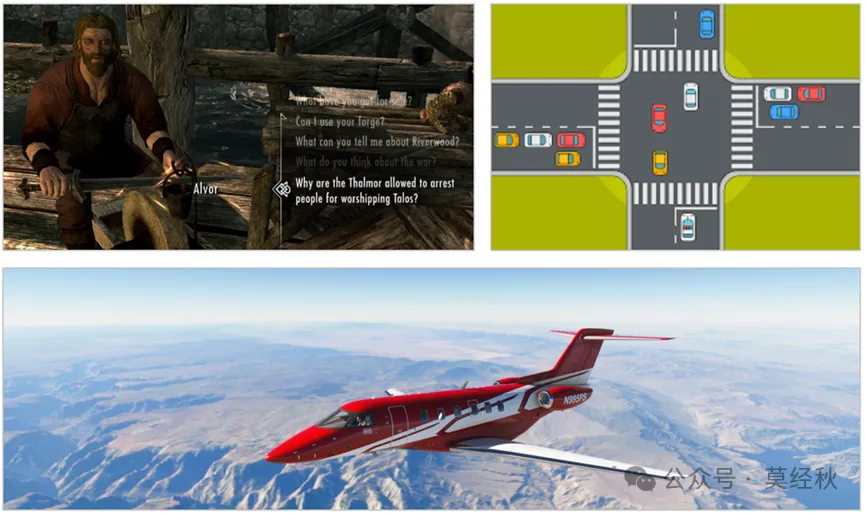
Various forms of AI Agents across different domains
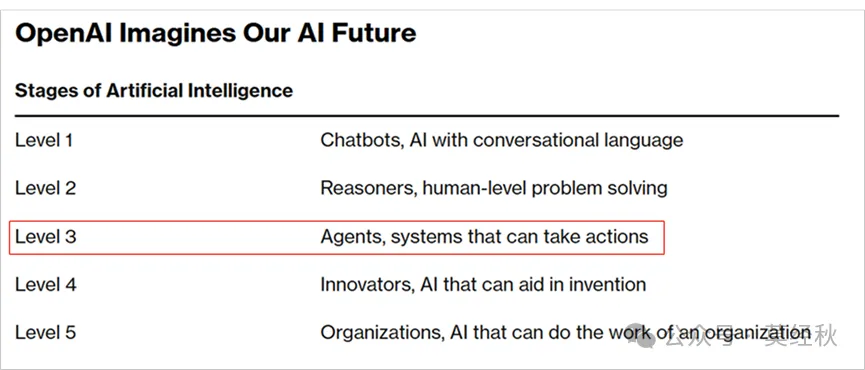
OpenAI's AGI development roadmap showing the progression towards advanced AI
The OpenAI AGI Roadmap
In OpenAI's AGI roadmap, AI development is divided into 5 levels:
Level 1 & 2
Level 1 represents chatbots that can understand and output information. Level 2 represents reasoning systems that can achieve human-level capabilities.
Level 3 - Agents
Level 3 represents systems that can take action - this is where agents come in, marking the widespread application of AI models.
Currently, ChatGPT represents the maturity of Level 1 AI, while models like OpenAI-o1 and DeepSeek-R1 demonstrate the realization of Level 2 reasoning capabilities. The next step for AI is to move from theory to practice through agents, finding carriers to deliver practical value.
Industry Perspective
From an industry perspective, agents as an application form are undoubtedly a necessary path for AI advancement. AI must achieve monetization through applications to secure resources for continuous evolution and improvement.
One could say that all AI model applications can be considered agents in a broad sense. This explains why ChatGPT is an agent, 360 Nano Search is an agent, Cursor's compilation interface is an agent, and even bank's intelligent voice sales representatives are agents.
Key Implications
- Agents bridge the gap between AI research and practical applications
- They represent the necessary step for AI commercialization
- The concept of agents encompasses a wide range of AI implementations
- Agents are crucial for AI's evolution from theory to practice
"AI remaining solely in papers and code cannot secure sufficient resources for continuous improvement. AI must realize value through applications, and consequently, agents as an application form are undoubtedly a necessary path for AI's advancement."
2. The Capabilities and Value Logic of Agents
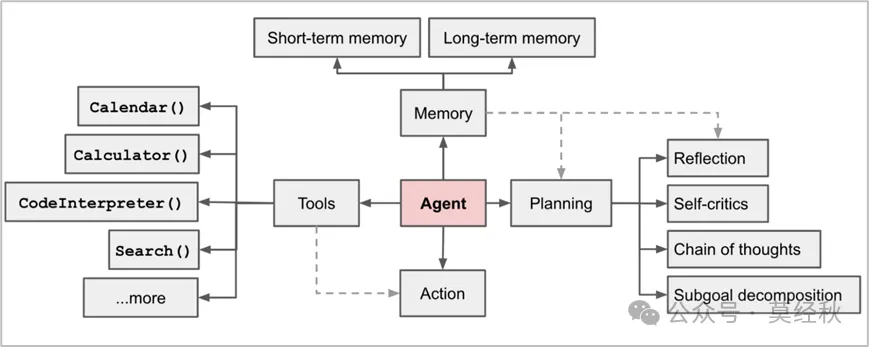
Lilian Weng's framework for agent capabilities
Evolution of Agent Capabilities
The definition of agent capabilities has evolved significantly over time. Initially, Lilian Weng, a former OpenAI expert, defined agents through four core components in her 2023 review:
Memory
Storage and retrieval of information across interactions
Planning
Strategic decision-making and task decomposition
Action
Execution of planned tasks and interactions
Tools
Utilization of external resources and capabilities
However, with recent advances in multimodal capabilities and reasoning abilities, this definition has shown its limitations. For instance, actions and tool usage are both output capabilities, and planning alone cannot fully describe an agent's reasoning and thinking abilities.
Modern Agent Architecture
Perception (Input)
- Multimodal understanding
- Image processing
- Audio analysis
- Video comprehension
- Environmental sensing
Control (Brain)
- Knowledge storage
- Chain-of-Thought reasoning
- Decision making
- Planning
- Context management
Action (Output)
- Text generation
- Tool utilization
- Physical interactions
- System operations
- Response formatting
Implementation and Workflow
In practice, a basic agent is a combination of different model nodes, logical components, and tool interfaces. For example, a search-based agent might require multiple steps:
- Initial search query processing
- Intent recognition
- Query reformulation
- Information source reduction
- Search results reranking
- Detail retrieval
- Content filtering
- Context enhancement
- Response generation
- Structured output formatting
The Unique Value Proposition
As we enter 2024, with advances in native multimodal models (like Gemini 2.0), tool-calling capabilities (like Anthropic Function Call), and reasoning models (like DeepSeek-R1), the question arises: Will agents become obsolete?
1. Complementing Model Limitations
- Enhanced reliability through multiple node control
- Improved precision through logical judgment components
- Human-in-the-loop collaboration capabilities
- Cost efficiency through small model orchestration
2. Extending Model Capabilities
- Integration with private knowledge bases via RAG
- Specialized multimodal model fusion
- Standardized tool chain integration
- Multi-agent system coordination
Case Study: ByteDance's AGILE Architecture
ByteDance demonstrated that 7B small models, when properly orchestrated in an agent architecture, can match or exceed the performance of 1800B models like GPT-4, offering a 100x cost advantage. This proves the unique value proposition of agent-based approaches in practical applications.
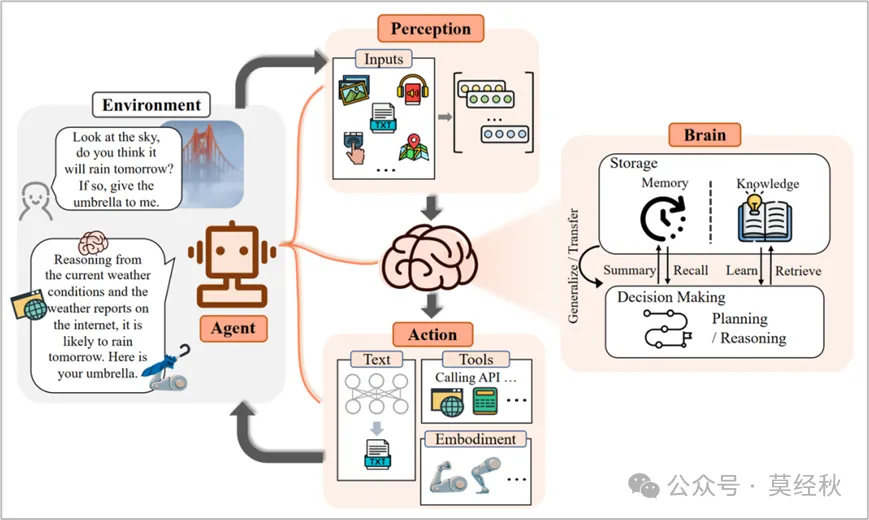
Fudan NLP Group's comprehensive framework for agent capabilities
3. A Classification System for AI Agents
Given the abstract nature of agents and their diverse forms, a clear classification system is essential for understanding the market and industry structure. This classification system considers both implementation complexity and application scenarios.
Primary Classification Dimensions
Agent Complexity
- Single Agent: Basic implementation with one model
- Agentic Workflow: Multiple nodes in sequence
- Multi-Agent System: Complex system with multiple coordinating agents
Task Complexity
- Tool-level: Simple, specific functions
- Process-level: Multi-step workflows
- Task-level: Complex problem-solving
General/Horizontal Agents
1. Chat Bots
- Examples: ChatGPT, Character.ai
- Complexity: Low (both agent and task)
- Features: Basic LLM with interface, emphasis on tool functionality
- Use Case: General conversation and information retrieval
2. Search Agents
- Examples: SearchGPT, 360 Nano Search
- Complexity: Medium (both agent and task)
- Features: Multi-node agent orchestration, process-focused
- Use Case: Enhanced search experiences with direct answers
3. Operator Agents
- Examples: OpenAI-Operator, AutoGLM
- Complexity: Medium (both agent and task)
- Features: Screen understanding, multimodal capabilities
- Use Case: Computer/phone/software control automation
4. Research Agents
- Examples: Microsoft-Magnatic One, Open-Deep-Research
- Complexity: High (both agent and task)
- Features: Complex multi-role agent orchestration, dynamic task planning
- Use Case: Autonomous research and analysis
Specialized/Vertical Agents
5. Content Agents
- Examples: Professional SD drawing applications, fine-tuned copywriting assistants
- Complexity: Low (both agent and task)
- Features: Direct model calls with domain specialization
- Use Case: Professional content generation
6. Copilots
- Examples: Cursor, Github-Copilot
- Complexity: Low (both agent and task)
- Features: Software plugin integration, specialized assistance
- Use Case: Professional workflow enhancement
7. Assistants
- Examples: BetterYeah, NICE-CX one
- Complexity: Medium (both agent and task)
- Features: Multi-node orchestration, business process integration
- Use Case: Enterprise customer service/marketing/workflow
8. Digital Labor
- Status: Emerging category, no prominent products yet
- Complexity: High (both agent and task)
- Features: Autonomous workflow planning and execution
- Use Case: Complete task automation without human intervention
Future Trends and Implications
As base models continue to evolve with enhanced multimodal and reasoning capabilities, the boundaries between agents and base models are becoming increasingly blurred. Many tasks that previously required complex agent orchestration can now be accomplished with a single base model.
However, this evolution doesn't diminish the value of agents. Instead, it pushes the agent ecosystem toward more specialized and sophisticated applications, particularly in:
- Enterprise-specific knowledge integration
- Complex workflow automation
- Multi-agent coordination systems
- Specialized domain applications