LLM Agents Research Breakthroughs in 2024
Table of Contents
- Understanding AI ROI Fundamentals
- Key Metrics for AI ROI Assessment
- Implementation Strategies and Best Practices
Introduction
As research into large language models (LLMs) deepens, experts have begun to reevaluate their capabilities. A recent Google study has highlighted that LLMs' planning abilities differ fundamentally from human cognition - while humans engage in detailed mental simulation, planning, and retrospection, LLMs merely predict the next token in a sequence.
Similarly, research from Arizona State University (ASU) has emphasized that LLMs cannot inherently perform planning and reasoning. Instead, they transform problems from one syntactic format to another symbolic representation, requiring external symbolic solvers for actual problem-solving.
Recently, Microsoft researchers have delved into the foundations of Agent AI, highlighting intelligent agents' capabilities in physical, virtual reality, mixed reality, and sensory interaction domains. They suggest that Agent AI could be key to the next generation of artificial intelligence. As LLM applications become increasingly complex, relying solely on language models presents significant challenges. Therefore, effectively leveraging LLM capabilities through AI agents appears to be a crucial development direction for this year.
Today, we present six cutting-edge research developments in LLM Agents for your reference and study.
DS-Agent

Traditional data processing and analysis heavily rely on professional data scientists, making it time-consuming and resource-intensive. By enabling LLM agents to act as data scientists, we can achieve more efficient insights and analysis while pioneering new industrial models and research paradigms. These specialized agents can autonomously process massive datasets, uncover hidden patterns and trends, provide clear model-building strategies with code, deploy models for inference, and create intuitive data visualizations.
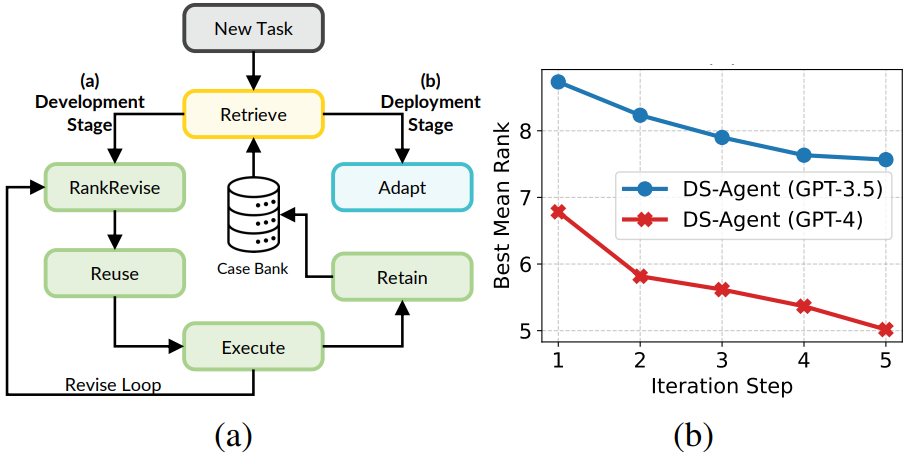
Recently, researchers from Jilin University and Shanghai Jiao Tong University introduced DS-Agent, an agent designed to handle complex machine learning modeling tasks. Technically, the team employed Case-Based Reasoning (CBR), a classic AI strategy, enabling the agent to leverage past experiences in solving similar problems.
LLM-Modulo

Perspectives on LLMs' planning and reasoning capabilities vary widely. Some are overly optimistic, believing appropriate prompting strategies are sufficient for these tasks. Others are pessimistic, viewing LLMs' only benefit as translating problems between syntactic formats, with actual problem-solving requiring external symbolic solvers.
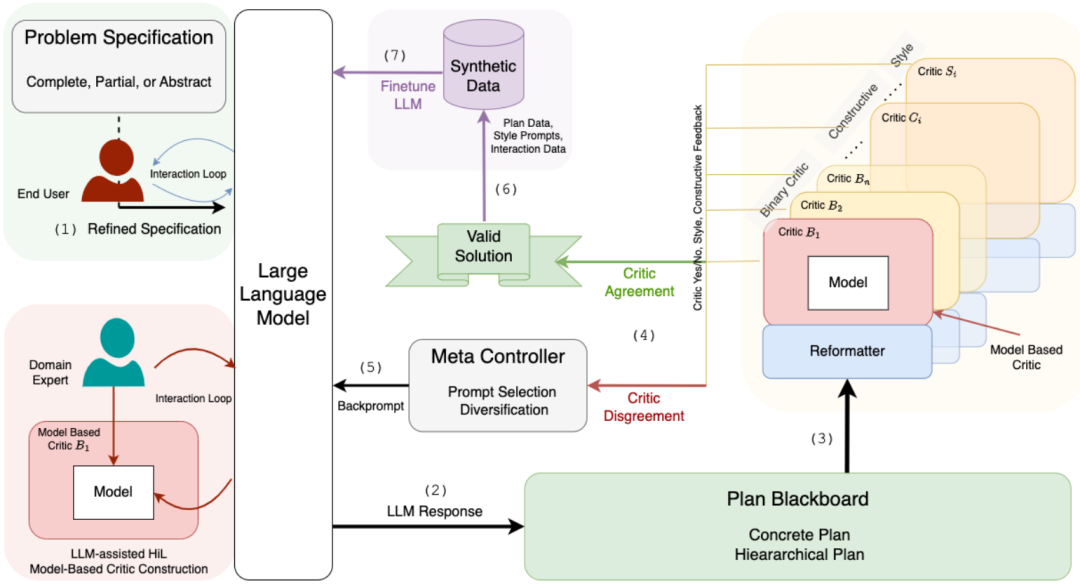
The authors' core argument is that while LLMs cannot independently perform planning and reasoning, they can play a crucial role in solving planning problems. They propose the LLM-Modulo framework, combining large language models with external verification tools to enhance LLMs' effectiveness in planning tasks.
SceneCraft

SceneCraft is an innovative LLM agent that converts textual descriptions into executable Python scripts for Blender, creating complex 3D scenes. It addresses spatial planning and layout complexities through high-level abstraction, strategic planning, and library learning.
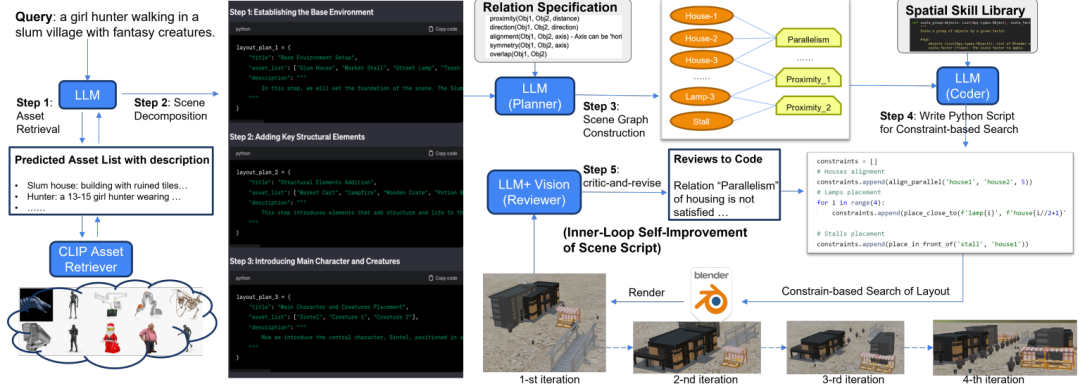
Specifically, SceneCraft first creates scene graphs, then writes scripts that transform spatial relationships into concrete numerical constraints. It utilizes vision-language models' perceptual capabilities to analyze and iteratively improve scenes. SceneCraft also features a library learning mechanism enabling self-improvement without LLM parameter adjustments. Evaluation results demonstrate SceneCraft's superior performance in rendering complex scenes and its potential applications in 3D scene reconstruction and video generation model control.
GitAgent

This research focuses on enhancing large language models like ChatGPT and GPT-4, particularly in handling complex tasks requiring multiple skills. While these models excel in language processing, their limited tool access often hinders their ability to address specialized user queries.
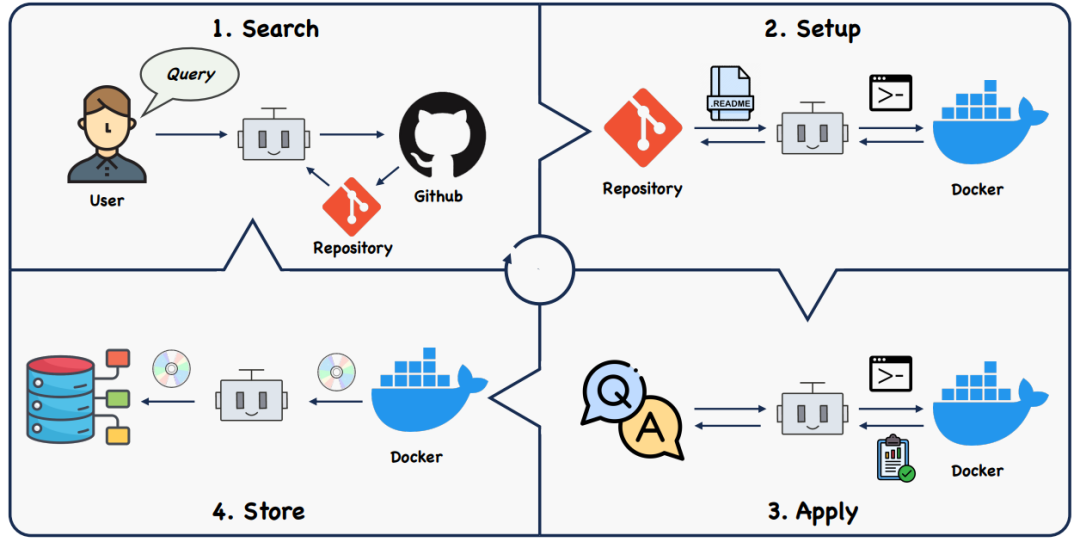
The authors developed GITAGENT, an agent that automatically discovers and integrates suitable code repositories from GitHub into its toolkit. GITAGENT operates in four phases and learns from GitHub solutions when encountering challenges. Experiments with 30 user queries demonstrated a 69.4% average success rate, validating the approach's viability.
LearnAct

While LLM Agents have gained significant attention, they face limitations in trial-and-error learning. This research emphasizes the importance of learning new actions from experience for enhancing LLM Agents' capabilities. Unlike humans who naturally expand their action space and skills through experiential learning, LLM Agents typically operate within fixed action spaces, limiting their growth potential.
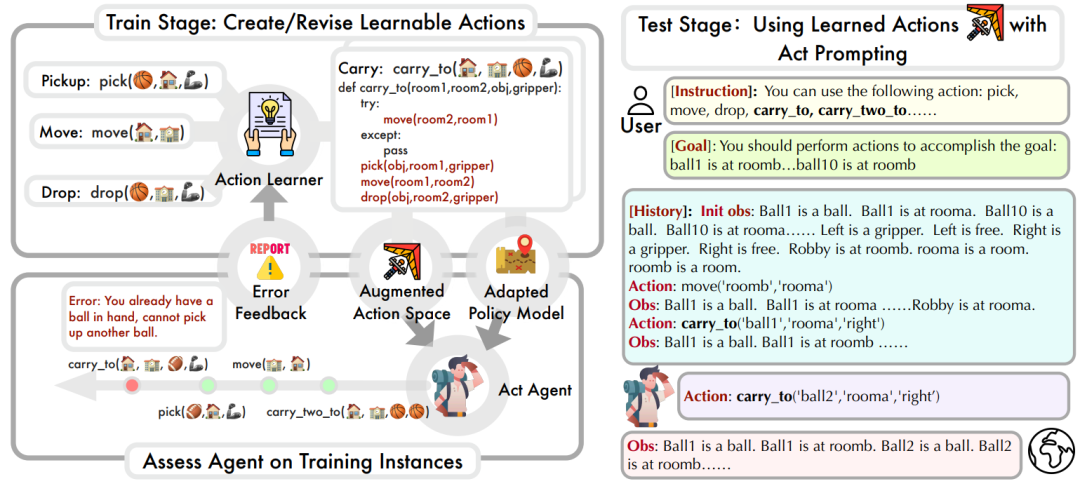
To address this challenge, the research introduces LearnAct, a framework employing iterative learning strategies to conduct open-ended action learning through Python function creation and improvement. During iteration, the LLM revises and updates actions based on failure experiences from training tasks to enhance effectiveness. Experimental evaluations in robot planning and Alfworld environments show that this open-ended action learning approach significantly improves agent performance on specific tasks after learning from just a few training task instances.
RepoAgent

Generative models have achieved remarkable success in software engineering, particularly in code generation and debugging tasks. However, their potential in automated code documentation generation remains largely untapped.
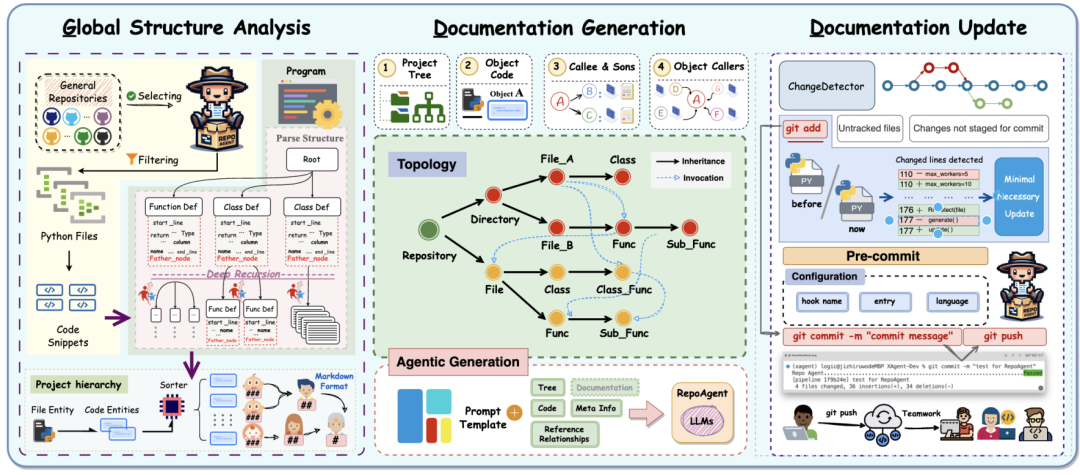
To bridge this gap, the authors developed REPOAGENT, an open-source framework based on large language models specifically designed for automatically generating, maintaining, and updating code documentation. Through a series of evaluations, including qualitative and quantitative analyses, they demonstrated REPOAGENT's capability in creating high-quality repository documentation.